How AI is improving financial fraud detection systems
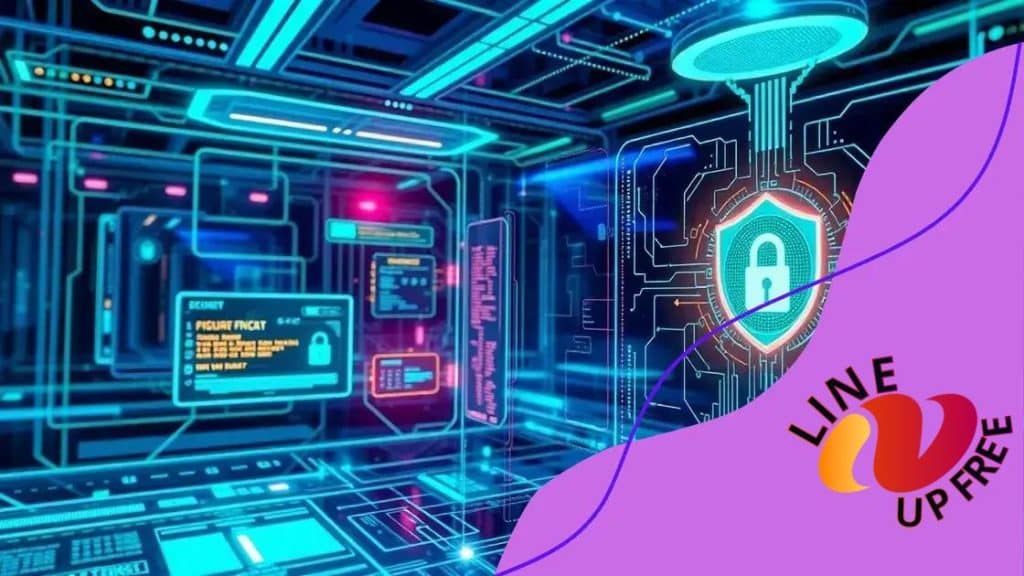
Anúncios
AI is significantly improving financial fraud detection systems by utilizing machine learning for real-time monitoring, advanced pattern recognition, and predictive analytics to identify and combat potential fraudulent activities efficiently.
How AI is improving financial fraud detection systems is a topic that’s gaining attention as financial institutions strive to enhance security. Have you ever wondered how technology evolves to outsmart fraudsters? Let’s explore this intriguing transformation.
Anúncios
Understanding financial fraud challenges
Financial fraud is a growing concern for organizations worldwide, affecting millions and costing billions annually. Understanding financial fraud challenges is crucial for developing effective prevention strategies. Fraudsters use sophisticated tactics to exploit vulnerabilities in systems, and recognizing these challenges is the first step to fortifying defenses.
Types of Financial Fraud
Different types of financial fraud can impact various industries. Here are some common forms:
- Credit Card Fraud: Unauthorized use of someone’s credit card information.
- Identity Theft: Stealing personal information to commit fraud.
- Account Takeover: Gaining control of a customer’s account to withdraw funds.
Every organization needs to be aware of these types. They not only damage finances but can harm reputations as well. The loss of customer trust can be significant.
Anúncios
Technological Vulnerabilities
The rise of digital transactions has made financial systems more vulnerable. Fraudsters leverage weaknesses in technology, such as:
- Phishing Attacks: Trick individuals into providing sensitive information.
- Malware: Infiltrating systems to gather data.
- Weak Authentication: Inadequate security measures for user access.
Understanding these vulnerabilities is essential for developing a robust fraud detection framework. Organizations must implement better security strategies to mitigate these risks.
Furthermore, the constant evolution of fraud tactics requires ongoing vigilance and adaptability. As fraudsters refine their strategies, businesses must stay ahead by adopting new technologies and enhancing their fraud detection systems.
In conclusion, addressing the challenges of financial fraud is a multifaceted effort. By recognizing the types of fraud, identifying technological vulnerabilities, and committing to ongoing improvements, organizations can better protect themselves and their customers.
The role of AI in transaction monitoring
Artificial intelligence (AI) plays a vital role in transaction monitoring, helping organizations to detect fraud efficiently and effectively. By analyzing vast amounts of data, AI can identify unusual patterns that human analysts might miss. This technology not only speeds up the detection process but also enhances overall security.
How AI Analyzes Transactions
AI systems use algorithms to assess transactions in real-time. These systems can learn from historical data, adjusting their models as they acquire new information. Some key aspects of how AI analyzes transactions include:
- Pattern Recognition: Identifying trends in transaction data to find anomalies.
- Machine Learning: Adapting to new fraud techniques without manual updates.
- Behavioral Analysis: Monitoring user behavior to spot deviations from typical patterns.
These capabilities make AI exceptionally suited for transaction monitoring. By continually analyzing data, AI systems can flag suspicious activities immediately, alerting financial institutions to possible fraud.
Benefits of Using AI
Integrating AI into transaction monitoring systems offers several advantages. It helps organizations reduce the risk of fraud while improving operational efficiency. Some benefits include:
- Increased Accuracy: Reducing false positives in fraud detection processes.
- Scalability: Handling larger volumes of transactions efficiently.
- Cost-Effectiveness: Lowering the costs associated with manual reviews.
By harnessing AI, organizations can build a more robust defense against financial fraud.
Furthermore, the integration of AI creates a proactive approach to security. Organizations that use AI can adapt more quickly to evolving threats, ensuring they remain a step ahead of fraudsters.
Machine learning techniques for fraud detection
Machine learning techniques are essential in enhancing fraud detection systems. These methods allow systems to learn from historical data and identify patterns indicative of fraudulent activity. By leveraging large datasets, organizations can improve their ability to detect fraud quickly and accurately.
Types of Machine Learning Techniques
Several types of machine learning techniques are employed in fraud detection, each serving distinct purposes. Understanding these methods is vital for effective implementation. Common techniques include:
- Supervised Learning: Models trained on labeled data that includes instances of fraud and non-fraud transactions.
- Unsupervised Learning: Used for anomaly detection, identifying outliers in transactions without prior labeling.
- Reinforcement Learning: AI learns optimal strategies through feedback from actions taken in fraud detection.
These techniques support the creation of models that can adapt to evolving fraud tactics and provide more accurate detection rates.
How Models are Trained
Training machine learning models for fraud detection involves several steps. First, historical transaction data is collected and prepared. This data is then categorized into fraudulent and legitimate transactions. Successful models depend on the quality of this data.
Once the data is prepared, the models are selected based on the type of learning chosen. After selection, the training process begins, where the model learns patterns associated with fraud. Regular updates and retraining are necessary because fraudulent tactics continuously evolve, requiring models to adapt.
Additionally, validating the model’s performance is crucial. Metrics such as precision and recall are analyzed to ensure the model accurately detects fraud while minimizing false positives. Balancing these metrics is a significant challenge in fraud detection and requires fine-tuning.
Real-world applications of AI in finance
Artificial intelligence (AI) is transforming the finance industry in numerous ways. Real-world applications of AI in finance are becoming increasingly evident as businesses adopt innovative technologies to enhance efficiency and security. These applications not only streamline operations but also improve customer experiences significantly.
Fraud Detection
One of the most prominent applications of AI is in fraud detection. Financial institutions leverage machine learning algorithms to analyze transaction data and identify patterns indicative of fraudulent activities. By processing transactions in real-time, AI systems can flag suspicious activities, dramatically reducing financial losses. The systems continuously learn from new data, improving their accuracy over time.
Risk Management
AI is also reshaping risk management practices. Through predictive analytics, organizations can assess financial risks more accurately. Using vast amounts of data, AI models can forecast potential risks by analyzing market trends, customer behavior, and economic indicators. This helps businesses make informed decisions, allocate resources effectively, and mitigate potential losses.
- Credit Scoring: AI algorithms evaluate creditworthiness by analyzing a wider range of data, leading to more fair and informed lending decisions.
- Portfolio Management: Robo-advisors use AI to create and manage investment portfolios, optimizing returns based on market conditions.
- Personalized Banking: Banks use AI to offer tailored services, understanding customer needs better and enhancing the overall banking experience.
Moreover, the integration of AI in personal finance management tools is growing. These tools help users budget and track spending patterns, providing personalized insights based on individual financial habits. The result is a more enlightened consumer that can make smarter financial choices.
The future of AI in finance looks promising, as continued advancements in technology will allow for even greater innovations. Businesses that embrace these technologies will lead in efficiency, security, and customer satisfaction.
Future trends in AI for fraud prevention
The landscape of fraud prevention is continuously evolving, and future trends in AI for fraud prevention are poised to reshape how organizations combat financial crimes. As technology advances, so do the strategies employed by fraudsters. This dynamic creates an ongoing need for enhanced fraud detection methods.
Enhanced Machine Learning Algorithms
In the near future, we can expect machine learning algorithms to become even more sophisticated. These advancements will enable systems to analyze data with greater precision and accuracy. Innovations in deep learning will empower AI to identify more subtle patterns that indicate fraud. This allows for real-time detection and response to suspicious activities.
- Predictive Analytics: AI will utilize predictive analytics to forecast fraudulent actions based on user behavior.
- Automated Learning: Systems will adapt on their own, improving efficiency without manual updates.
- Integration of AI with Blockchain: Combining AI and blockchain technology can help create secure transaction records that are immutable.
As AI technologies continue to progress, organizations will be better equipped to handle evolving fraud tactics.
Real-Time Monitoring and Response
Another significant trend is the shift toward real-time monitoring and immediate response. Businesses will increasingly invest in systems that can flag and address potential fraud while it is occurring. These innovations will involve the use of AI-driven insights to identify irregularities as they arise.
By implementing real-time analytics, organizations can not only improve their fraud detection processes but also enhance customer trust. Customers will feel more secure knowing that their transactions are actively monitored for signs of fraud. This proactive approach is crucial in maintaining competitiveness in the finance industry.
Additionally, companies will focus on building user-friendly interfaces that facilitate easy reporting of suspicious activities by consumers. This engagement will create a collaborative environment in the fight against fraud.
FAQ – Frequently Asked Questions about AI in Fraud Prevention
How does AI help in detecting fraud?
AI analyzes large amounts of transaction data to identify patterns and anomalies that may indicate fraudulent activity.
What are the benefits of real-time monitoring?
Real-time monitoring allows organizations to respond quickly to suspicious activities, reducing potential financial losses.
How do machine learning algorithms improve fraud detection?
Machine learning algorithms continuously learn from new data, refining their ability to detect fraud as tactics evolve.
Can AI enhance customer trust?
Yes, by effectively preventing fraud, AI helps build customer confidence in financial institutions, leading to stronger relationships.