How machine learning is improving supply chain management
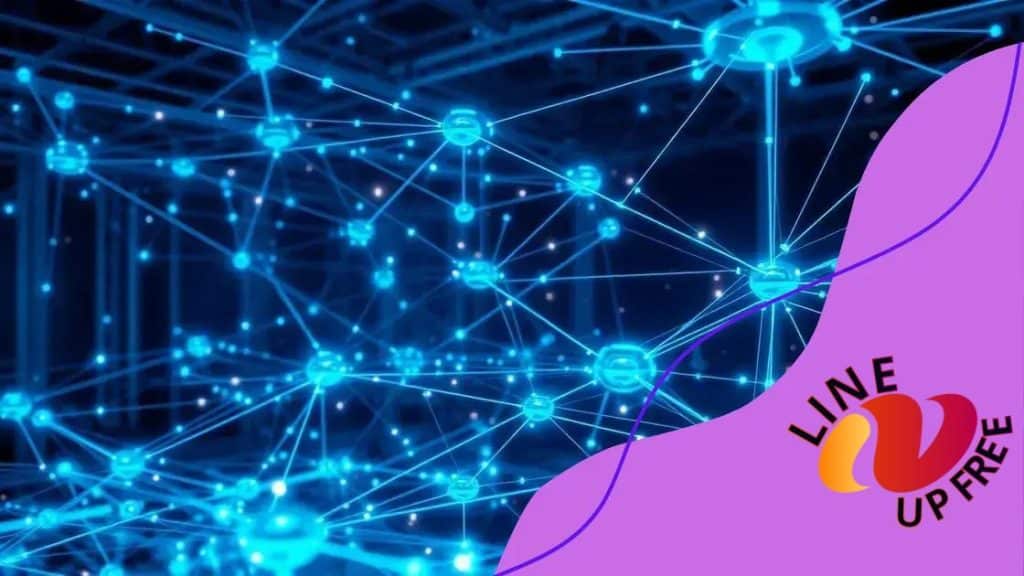
Anúncios
Machine learning is transforming supply chain management by increasing efficiency, improving forecasting accuracy, optimizing logistics, and promoting sustainability, while also presenting challenges such as data quality, integration, and ethical considerations.
Isn’t it fascinating how machine learning is reshaping industries? In supply chain management, this technology offers new ways to optimize processes and make smarter decisions. Let’s dive into how this works and what it means for businesses.
Anúncios
Understanding the basics of machine learning
Understanding the basics of machine learning is essential for grasping how it enhances various industries, including supply chain management. This technology mirrors how humans learn from experience, enabling systems to improve over time without explicit programming.
What is Machine Learning?
At its core, machine learning involves algorithms that analyze data, recognize patterns, and make decisions. By processing vast amounts of information quickly, these algorithms can identify trends that would otherwise go unnoticed.
Key Types of Machine Learning
Machine learning is categorized mainly into three types:
Anúncios
- Supervised Learning: This involves training a model on labeled data, allowing it to make predictions.
- Unsupervised Learning: In this type, the model analyzes and groups unlabelled data based on similarities.
- Reinforcement Learning: Here, an agent learns by interacting with its environment and receiving feedback.
Each type plays a significant role in how machine learning impacts supply chains. For example, supervised learning can forecast demand, while unsupervised learning helps in segmenting customers based on purchasing behavior.
How Machine Learning Works
Machine learning employs various techniques to process data. One common method is through neural networks, which mimic how the human brain processes information. These networks consist of interconnected nodes that work together to derive insights from data.
Another important technique is decision trees, which help outline the decision-making process in a clear, visual format. This makes it easier for businesses to understand the possible outcomes of different choices.
The dynamic nature of machine learning means that as more data becomes available, the systems can adapt and improve their performance. This is particularly valuable in supply chain management, where the ability to respond quickly to changes can result in significant cost savings and improved efficiency.
Key benefits of machine learning in supply chains
The key benefits of machine learning in supply chains are transforming the way businesses operate. By effectively analyzing data, organizations can enhance their operations and decision-making processes significantly.
Increased Efficiency
One of the main benefits of machine learning is increased efficiency. Algorithms can process vast amounts of data quickly, allowing for faster decision-making. This reduces the time spent on manual tasks.
Improved Forecasting
With machine learning, companies can improve their forecasting accuracy. Historical data patterns are analyzed to predict future demand, which minimizes stockouts and overstock situations.
- Enhanced Demand Planning: Better insights help align production with actual customer demand.
- Real-Time Adjustments: Automated adjustments can be made based on live data inputs.
- Cost Savings: Reduced inventory costs lead to significant savings.
In addition to improving forecasts, machine learning can optimize logistics. By analyzing transportation routes and methods, businesses can reduce delays and costs. This data-driven approach ensures that resources are used effectively.
Risk Management
Another critical advantage of machine learning in supply chains is enhanced risk management. By identifying potential risks early, companies can take proactive measures to mitigate them. This real-time analysis helps businesses make informed decisions during disruptions.
Moreover, machine learning algorithms can analyze market trends, allowing companies to adapt their strategies quickly. This agility is vital in today’s rapidly changing environment, helping businesses stay ahead of competitors.
Furthermore, using machine learning enhances customer satisfaction. By providing accurate delivery estimates and improving service quality, companies build stronger relationships with their customers.
Real-world applications of machine learning
Real-world applications of machine learning are wide-ranging and impactful across various industries. Businesses are leveraging this technology to streamline operations and enhance decision-making.
Healthcare Innovations
In healthcare, machine learning plays a crucial role in diagnostics and patient care. Algorithms analyze medical records and images to detect diseases earlier and more accurately.
Supply Chain Optimization
Within supply chains, machine learning is used to optimize inventory management and logistics. For example, predictive analytics help forecast demand, reducing waste and ensuring products are available when needed.
- Demand Forecasting: By analyzing past sales data, businesses can predict future buying patterns.
- Route Optimization: Machine learning algorithms find the most efficient delivery routes.
- Inventory Management: Automated systems ensure the right stock levels are maintained.
Apart from logistics, machine learning enhances quality control and customer service in the supply chain. Businesses can identify defects more quickly, saving time and money.
Finance and Banking
In finance, machine learning is transforming how companies assess risks and detect fraud. Algorithms analyze transaction data to spot unusual behaviors that may indicate fraudulent activity.
This technology also supports credit scoring, allowing lenders to determine borrower risk more accurately by evaluating a broader range of factors. As a result, better financial decisions can be made.
Marketing Strategies
Another key area is marketing, where machine learning allows businesses to tailor their campaigns. By analyzing consumer data, companies can create personalized experiences for their customers.
These applications can include targeted advertising and recommendation systems that suggest products based on previous purchases and browsing history. This approach significantly enhances customer engagement and satisfaction.
Challenges faced in implementing machine learning
Implementing machine learning comes with several challenges that organizations must navigate carefully to succeed. Understanding these challenges is essential for anyone looking to adopt this powerful technology.
Data Quality Issues
One of the primary challenges is ensuring the quality of data. Machine learning algorithms require large amounts of accurate and clean data to function effectively. Poor data quality can lead to incorrect outputs and unreliable results.
Integration with Existing Systems
Another significant hurdle is integrating machine learning solutions with existing systems. Companies often have legacy systems that may not be compatible with new technologies. This integration can require substantial time and resources.
- Compatibility Testing: Systems must be tested to ensure they work together.
- Training Staff: Employees may need training to adapt to new tools.
- Resource Allocation: Companies must allocate resources to manage this transition.
Additionally, organizations often face resistance to change among employees. People may feel uncertain about how machine learning will impact their jobs, leading to reluctance in adopting new methods.
Ethical Considerations
Ethics is another important concern. Machine learning systems can potentially introduce bias into decision-making processes. If the training data reflects existing biases, the algorithms could perpetuate these issues, leading to unfair outcomes.
Ensuring transparency in how algorithms make decisions is also crucial. Organizations need to communicate clearly about how machine learning is used and how decisions are made.
Cost and Resource Demands
The cost of implementing machine learning solutions can be substantial. Businesses must consider the financial investment required for technology, data storage, and talent acquisition.
Hiring skilled personnel is essential, as data scientists and machine learning experts command high salaries. Without the right talent, even the best technologies may not be utilized effectively.
Future trends in supply chain management
The future of supply chain management is evolving quickly, driven by advancements in technology and innovative practices. As machine learning and data analytics become more integrated, companies are discovering new ways to enhance their operations.
Increased Automation
One key trend is the increased automation of supply chain processes. Automation technologies like robotics and AI are streamlining operations, reducing manual work. This shift allows employees to focus on higher-level tasks while machines handle repetitive activities.
Data-Driven Insights
Another trend is the rise of data-driven insights. As organizations gather more data, they can analyze it to make informed decisions. Utilizing predictive analytics, companies can forecast demand with greater accuracy, improving inventory management and reducing waste.
- Smart Inventory Management: Real-time data helps in determining the right stock levels.
- Dynamic Pricing Models: Prices can adjust based on current market conditions.
- Customized Supply Chains: Tailoring logistics based on customer data enhances satisfaction.
In the future, supply chains will rely heavily on interconnected systems. This connectivity allows for seamless information flow between suppliers, manufacturers, and distributors. Such integration leads to quicker responses to market changes and unexpected events.
Sustainability Efforts
Sustainability is becoming a vital consideration in supply chain management. Businesses are focusing on reducing their carbon footprints and implementing more eco-friendly practices. This shift is not just good for the planet; it’s also good for business, as consumers increasingly prefer sustainable products.
As organizations adopt sustainable practices, they often invest in renewable energy sources and eco-friendly packaging. This helps them meet both regulatory requirements and customer expectations, enhancing their brand image.
Collaboration and Transparency
Collaboration across the supply chain is another future trend. As companies recognize the value of partnerships, they are working together to optimize their supply chains. Transparency is crucial here, as consumers demand to know where products come from and how they are made.
Technologies like blockchain are being explored to improve transparency. This decentralized ledger can provide an immutable record of products’ journeys through the supply chain, enhancing trust among consumers and businesses alike.
In conclusion, the integration of machine learning into supply chain management brings substantial benefits along with specific challenges. As technology advances, we can expect increased automation, better data-driven decisions, and enhanced sustainability practices. However, organizations must navigate concerns such as data quality and ethical considerations to fully harness these advantages. By embracing collaboration and transparency, companies can position themselves for success in an increasingly competitive landscape.
FAQ – Frequently Asked Questions about Machine Learning in Supply Chain Management
What is the main benefit of using machine learning in supply chains?
The main benefit is increased efficiency, as machine learning helps optimize processes, forecasts demand accurately, and improves inventory management.
How does data quality affect machine learning outcomes?
High-quality data is crucial because poor data can lead to inaccurate predictions and unreliable results in machine learning algorithms.
What challenges do companies face when implementing machine learning?
Companies face challenges such as integration with existing systems, data quality issues, and ethical considerations, including bias in decision-making.
How can sustainability be integrated into supply chains using machine learning?
Machine learning can help identify areas for efficiency and waste reduction, enabling companies to adopt more sustainable practices in their supply chains.