How machine learning is transforming education data analysis
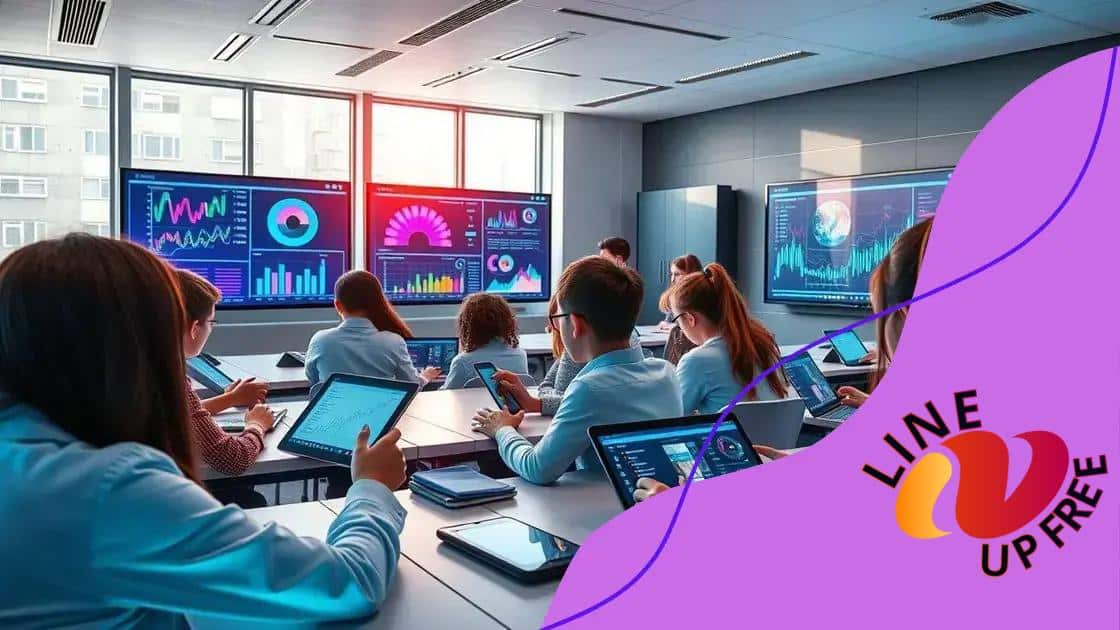
Anúncios
Machine learning is transforming education data analysis by enabling personalized learning, enhancing decision-making, and providing real-time insights into student performance, while also presenting challenges like data privacy and resource limitations.
How machine learning is transforming education data analysis is crucial for shaping the future of teaching and learning. Have you ever wondered how data can enhance classroom experiences? In this article, we explore the implications of these technologies.
Anúncios
Understanding machine learning in education
Understanding machine learning in education is essential for harnessing the power of data to improve teaching methods and student outcomes. This innovative technology allows educators to analyze vast amounts of information, identifying patterns and insights that can lead to better learning experiences.
Anúncios
What is machine learning?
Machine learning is a subset of artificial intelligence (AI) that enables systems to learn from data and make predictions. By using algorithms, these systems can adapt and improve over time, which is particularly valuable in educational settings.
Key features of machine learning in education
- Personalized learning: Tailoring educational content to meet the needs of individual students.
- Data-driven insights: Utilizing data analysis to guide instructional strategies and improve curriculum.
- Adaptive assessments: Adjusting tests and evaluations based on student performance in real-time.
- Predictive analytics: Forecasting student outcomes and identifying those who may need additional support.
With the help of machine learning, educators can enhance their understanding of student engagement and performance. By analyzing various factors, including attendance, participation, and grades, schools can create tailored interventions that support at-risk students. This approach fosters a more equitable learning environment.
Moreover, machine learning technologies are used to develop intelligent tutoring systems that provide personalized feedback to learners. These tools adapt to each student’s pace, ensuring that no one falls behind. Educators can also benefit from these insights to refine their teaching methods and strategies.
Real-world examples of machine learning in classrooms
Many institutions are already experiencing the benefits of machine learning. For instance, universities are employing analytics to track student performance across courses and intervene before students face major challenges. Secondary schools are using data to tailor lessons to improve engagement effectively. This initiative promotes active participation and ensures that students remain focused on their studies.
As technology continues to evolve, the potential for machine learning in education will grow exponentially. By embracing these tools, educational institutions can transform their approaches to teaching, ultimately leading to better student outcomes and a more informed educational landscape.
Key benefits of data analysis in learning
The key benefits of data analysis in learning are reshaping educational practices and providing valuable insights for educators. By effectively analyzing data, schools can enhance teaching strategies and improve student outcomes.
Improved decision-making
Data analysis allows educators to make informed decisions based on actual performance metrics. Understanding how students learn helps teachers adapt their lessons to meet diverse needs. When schools utilize data, they can pinpoint areas that require focus and adjustments.
Personalized learning experiences
With insights gained from data, educators can create personalized learning plans for each student. This approach acknowledges different learning styles and paces. By closely monitoring progress, teachers can intervene promptly and ensure that every student receives the support they need.
- Identifying strengths and weaknesses: Data can reveal what subjects a student excels in and where they struggle.
- Tailoring resources: Teachers can provide specific resources tailored to each student’s needs.
- Facilitating engagement: Customizing learning paths keeps students engaged and motivated.
Moreover, data analysis fosters collaboration among educators. Sharing insights about student performance can lead to collective efforts in improving teaching methods. For instance, when teachers work together on data-driven decisions, they can develop effective strategies to support at-risk students.
Enhanced tracking and accountability
Through data analytics, schools can continuously track student progress and evaluate the effectiveness of academic programs. This information holds educational institutions accountable while providing transparency for parents and stakeholders. Reporting data contributes to fostering trust within the community.
Additionally, using data to measure success fosters a culture of continuous improvement. Educators become more aware of the impact of their methods, leading to ongoing refinements in curriculum delivery. Feedback from data analysis is invaluable for achieving educational goals.
The integration of data analysis into educational practices paves the way for innovations. As schools embrace technology and analytics, they can create a more responsive learning environment. This evolution enhances not just academic performance but also cultivates a love for learning among students.
Real-world applications of machine learning
The real-world applications of machine learning in education are transforming the way we teach and learn. From tailored learning paths to smarter administrative processes, machine learning is revolutionizing the educational landscape.
Personalized Learning Programs
One major application is developing personalized learning programs that adapt to individual student needs. By analyzing student data, machine learning algorithms can identify the best learning materials and strategies for each student. This individualized approach helps keep students engaged and supports their unique learning styles.
Intelligent Tutoring Systems
Another exciting application is intelligent tutoring systems. These systems provide real-time feedback and guidance to students as they work through problems. The AI analyzes responses and adjusts difficulty levels, ensuring students are challenged without feeling overwhelmed. This method promotes a better understanding of concepts.
- Automated grading: Machine learning can speed up the grading process, allowing teachers to focus more on instruction.
- Learning analytics: Educators can use insights derived from data for real-time monitoring of student performance.
- Early warning systems: Identifying students who may be at risk of falling behind helps educators intervene more effectively.
Furthermore, machine learning enhances administrative efficiency in schools. For example, it can help streamline admissions processes by analyzing applications and predicting student success. Schools can also use machine learning for scheduling classes, optimizing resource allocation, and improving overall operation.
As we continue to embrace technology, the impact of machine learning on education will only grow. Schools that successfully implement these systems can expect improvements in both student achievement and operational efficiency. Staying informed about these advancements is key to fostering a modern educational environment.
Challenges in implementing machine learning
The challenges in implementing machine learning in education are significant and must be addressed to maximize its potential. While this technology holds great promise, several obstacles can hinder its effective integration into classrooms.
Data Privacy Concerns
One major challenge is ensuring data privacy and security. Schools handle sensitive student information, and using this data for machine learning can raise concerns about privacy breaches. It’s crucial for educational institutions to establish strong data protection policies that comply with regulations.
Infrastructure Limitations
Another hurdle is the need for adequate infrastructure. Many schools may lack the necessary hardware and software to support machine learning applications. This limitation can prevent them from fully leveraging the benefits of technology in their teaching and administrative processes.
- Cost barriers: Implementing machine learning can be expensive, particularly for underfunded schools.
- Integration challenges: Existing systems may not easily incorporate new technologies, creating obstacles in data flow.
- Technical expertise: Teachers and staff may require additional training to effectively use machine learning tools.
Additionally, machine learning solutions can sometimes produce biased results if not trained on diverse datasets. This may lead to unfair treatment of certain student groups, which is a significant concern for educators. Ensuring that machine learning models are trained on comprehensive data is essential for equity in the classroom.
Moreover, a lack of clear understanding among stakeholders about how machine learning can aid education can hinder its acceptance. Educators must be informed and supported to embrace these technologies fully. Building a culture of innovation and continuous learning is key to overcoming resistance and maximizing the benefits of machine learning.
As educational institutions gradually adopt machine learning, addressing these challenges will be vital for success. By creating supportive environments that prioritize data privacy and infrastructure improvements, schools can better harness the advantages of technology in education.
Future trends in educational data analysis
The future trends in educational data analysis are shaping the way institutions will enhance learning experiences and outcomes. As technology continues to evolve, several key trends are emerging that promise to make education more data-driven.
Integration of Artificial Intelligence
One significant trend is the integration of artificial intelligence (AI) in educational data analysis. AI can automate data collection and analysis processes, providing real-time insights into student performance. This shift allows educators to focus more on instruction and less on data management.
Increased Use of Predictive Analytics
Predictive analytics is another promising trend. By analyzing historical data, predictive models can identify at-risk students before they fall behind. This proactive approach enables educators to intervene early, providing targeted support for those who need it most.
- Enhanced learning analytics: Schools will increasingly use data to understand the complete learning journey of each student.
- Adaptive learning systems: These systems will personalize learning experiences based on student data and preferences.
- Data collaboration: Institutions will collaborate on shared data platforms to benchmark and improve educational strategies.
Moreover, the evolution of data visualization tools will make it easier for educators and administrators to interpret complex data sets. Intuitive dashboards will allow for quick access to key performance indicators (KPIs), leading to faster decision-making in curriculum adjustments.
As we look ahead, the role of data ethics will become increasingly important. Educational institutions will need to ensure that data is used responsibly and that student privacy is protected. Building trust with parents and students will be essential in this data-driven future.
The continuous advancement in data analysis technology will empower educators and institutions, ultimately creating a more effective and personalized educational environment. Embracing these trends will be key to enhancing student success and facilitating lifelong learning.
FAQ – Frequently Asked Questions about Machine Learning in Education
How does machine learning enhance personalized learning in classrooms?
Machine learning analyzes student data to create customized learning experiences, allowing educators to tailor instruction based on individual needs.
What are the key benefits of using data analysis in education?
Data analysis helps improve decision-making, facilitates personalized learning, tracks student progress, and holds educational institutions accountable.
What challenges might schools face when implementing machine learning?
Challenges include data privacy concerns, inadequate infrastructure, the need for technical expertise, and potential biases in data.
What future trends should educators be aware of regarding educational data analysis?
Future trends include integration of AI, increased use of predictive analytics, enhanced data visualization tools, and a focus on data ethics.